The new road ahead
AD technology is manifested in robotaxis, passenger cars, and heavy goods vehicles. But they all follow distinct development pathways and face unique motivations and challenges.
9 min read
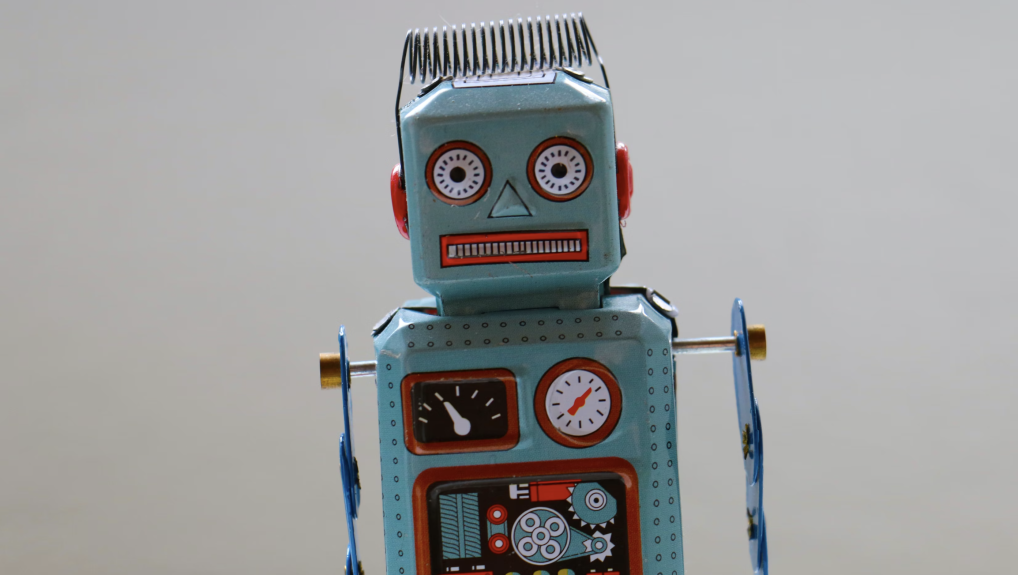
So far, robotaxis have shown the way to full, unsupervised automation. Waymo was the first to get the green light to run its driverless taxi service in California and quickly began charging for rides in San Francisco. GM’s Cruise also received the California Public Utilities Commission’s approval to operate their driverless taxis around the clock in the state.
Despite struggling with weird-looking objects, blocking lanes, and obstructing other vehicles (sometimes, however, with dramatic consequences*), self-driving taxis have had an overall successful launch, considering the impressive number of incident-free hours of driving so far. They rightly promise affordable, safe, and scalable transportation services while offering a decent chance of reducing traffic congestion, easing parking issues, and lowering pollution and energy use – at least if they use electric vehicles. They can also improve transportation access for people without cars or who can’t drive. In short, robotaxis are likely here to stay, and cities will surely open themselves to them just like they did to fossil-driven cars a century ago.
Evolving paths in vehicle autonomy
Initially, Robotaxi and personal car AD technologies evolved along different paths, particularly in their sensor configurations. They began to converge for a while as the sensor technologies used in both became more similar. However, they have recently started to diverge once again. Moreover, robotaxis increasingly rely on remote operations to resolve issues the vehicle cannot handle itself (remote control isn’t necessary for “standard” operations/situations). Currently, there’s talk of one remote operator for every ten cars, and while this might not be prohibitively expensive, it does add a significant additional cost.
Now, consumer vehicles also mainly emphasize personal benefits. Here, enhanced safety (from reduced human errors) and the luxury of multitasking stand out; a self-driving car frees up time for the user, offers safe driving, and, of course, cool new technology for the owner to show off. It’s unclear, however, if and how autonomous passenger vehicles will have a net positive climate impact. Indeed, despite automation promising safety, convenience, and energy-efficient driving, the environmental sustainability of data-hungry systems is uncertain, urging efficient data management strategies and regulatory intervention. If you also factor in increasingly lower prices, which make autonomous vehicles (AVs) more affordable and accessible, their usage will likely increase even more.
Automated heavy goods vehicles primarily focus on economic and efficiency motivations. For this industry, automation means reducing labor costs and increasing transport efficiency for industries reliant on transportation, like logistics. Autonomous trucks could operate around the clock, barring maintenance and refueling stops, making goods transportation faster and more predictable. One can also argue that professional drivers are not always skilled drivers. Just like ordinary civilians behind the wheel, they too can become tired and stressed and take risks they shouldn’t. Such examples are not hard to find among truck and taxi drivers.
Navigating challenges
Okay, so robotaxis are here. That’s all well and good. But why do consumer cars take so long to hit the streets with extended self-driving capabilities? Why are ordinary passenger cars only allowed to drive autonomously on limited stretches on certain highways, in a few countries, and at a very moderate pace?
The development and deployment of advanced self-driving technology in consumer cars have been slower than some might expect for various reasons, many of which revolve around safety, regulatory challenges, and technological limitations. Self-driving technology must be incredibly safe to be accepted by the public and to satisfy regulators. While robotaxis can be monitored and controlled by a central system and are usually confined to well-mapped and studied areas, consumer cars need to be able to handle a much more comprehensive range of driving scenarios.
To get to that point, the software must be trained on various driving scenarios, from basic to highly complex traffic situations. This includes environmental traffic conditions such as weather, darkness, and road quality and understanding human driving patterns. You can analyze any traffic scenario to pinpoint how accidents, incidents, and near-misses occur by amassing extensive amounts of real-life traffic information. This information can then be used to develop software that creates safe margins for vehicles, pedestrians, or other moving or stationary objects.
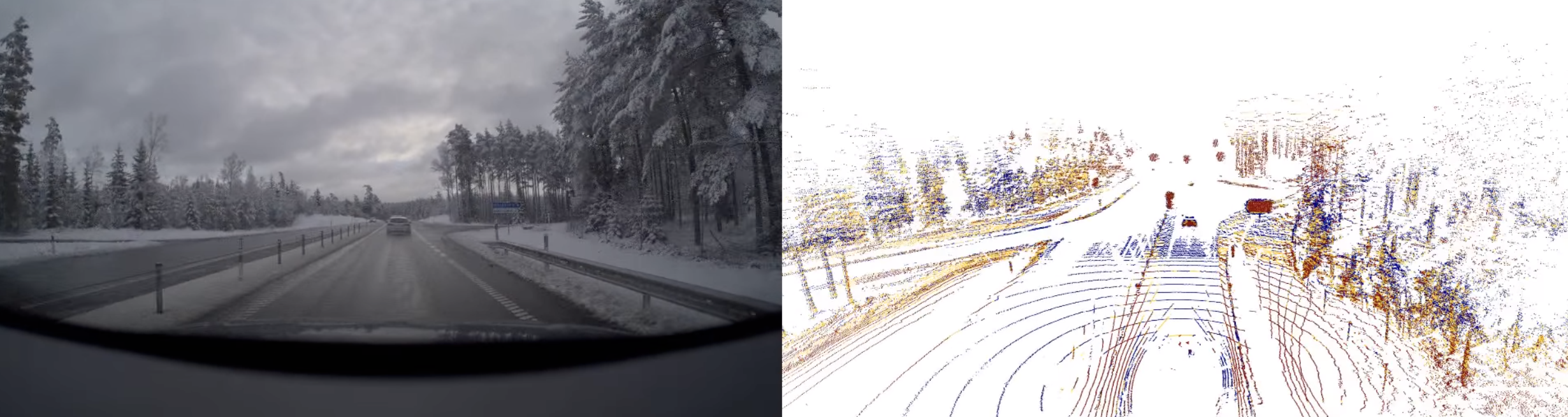
Simply put, a consumer vehicle needs to be trained on every corner of the world, whereas a robotaxi has wildly expensive equipment and is trained on a limited environment. A passenger car must be able to handle Place d’Étoile in Paris, lane-changing on the Autobahn at 150 km/hour, and dark, slippery, snowy Nordic winter roads. And even though significant advances have been made, self-driving technology still has limitations, such as understanding nuanced human behaviors on the road. But reasonably (and it’s also to our advantage), those of us working on ‘consumer vehicle automation’ can, for now, exclude the most challenging tasks since we still have a capable human driver on board. However, in the long run, it’s true that automation offerings will apply to all driving. But we don’t need to do that to have a functional and marketable product at the moment.
Moreover, the regulations surrounding self-driving cars are complex and still under development in many areas. Laws and regulations must be established for how the cars operate and how they interact with other road users, how they should respond in emergencies, and so forth. Because of these and other challenges such as infrastructure, ethical and legal questions, and data and privacy issues, the introduction of self-driving capabilities in consumer vehicles has been more incremental, often starting with features like lane-keeping assistance, adaptive cruise control, and parking assistance as stepping stones toward full autonomy.
To be sure, the development and adoption of robotaxis also need to improve. For one, there are regulatory complexities, with the need for clear policies addressing the operation of vehicles without human drivers. These regulations invariably touch on safety and liability concerns. Additionally, robotaxis rely heavily on city infrastructure and may require specialized traffic management or vehicle-to-infrastructure communication systems. It isn’t easy to achieve – and prove – sufficient safety and handle practical challenges when you need to handle such a high volume of rides from A to B.
Also, winning public trust remains challenging, mainly since accidents and malfunctions draw so much media attention. And what about all the tasks that taxi drivers typically perform? They clean their car, assist people with physical or cognitive issues, carry luggage, ensure passengers remember their belongings, etc.
One goal, many obstacles
Autonomous passenger cars grapple with partly the same sets of challenges. Most of our current infrastructure is built around human drivers. Autonomous vehicles may require upgrades in road signs, traffic signals, and even the design of intersections to operate optimally. Importantly, like robotaxis, consumer cars must navigate in mixed traffic, where non-autonomous vehicles introduce unpredictable behaviors.
What’s more, convincing the public to trust a machine with their safety is a significant hurdle. Any high-profile accidents or malfunctions can severely damage public confidence. AVs will also record everything around them, generating vast amounts of data that should be managed sustainably. Ensuring that this data is secure and not misused poses yet another challenge. Although achieving unsupervised automation is challenging – not to mention proving its safety – limiting the operational design domain (ODD) can ease these requirements.
Heavy goods vehicles face a blend of these challenges. Like robotaxis, they require stringent regulatory clearance given the potential risk of large vehicles on highways. And like passenger cars, they need advanced systems to interact safely with other road users.
However, they also face industry-specific issues, such as managing long-haul logistics with automated systems or ensuring secure unloading in varied environments. To truly benefit from automation, they need to be able to drive the entire way from point A to point B without reloading. And that’s a large ODD, larger than what robotaxis handle. Otherwise, you might still need to have a human on board.
Counting the cost
Robotaxis demand a high initial investment. This includes, among other things, research and development, regulatory approvals, and the procurement of an initial fleet. However, as the service scales, there’s the potential for achieving decent returns on the investment; as fleets grow, the cost per vehicle could decrease. Furthermore, the shared-use model means vehicles have a higher utilization rate, which can accelerate the return on investment.
In the realm of passenger cars, integrating autonomous systems introduces additional costs. The sensors and computing equipment required for reliable self-driving are still relatively expensive. Although costs are coming down, outfitting consumer cars with this technology can add a significant amount to the price, which could limit mass adoption. Manufacturers must also consider the ongoing costs of maintaining and updating these systems, especially as technology evolves and regulations change.
For commercial vehicles, the initial investment in automation technology can be justified by potential long-term savings. Reduced labor costs, combined with increased efficiency and reduced downtime, can make the financial case for autonomy in the commercial sector compelling. The automated truck doesn’t need to rest like a human driver does. This could double the utilization rate or even more.
The road to scalability
Robotaxis typically follow a city-by-city deployment strategy. They begin by establishing a presence in specific cities or areas, then expand as they navigate and address regulatory and infrastructural challenges. Managing a robotaxi fleet also requires robust systems for maintenance, charging (especially for electric fleets), and monitoring vehicle health.
Autonomous passenger cars have the advantage of a potentially global market. However, different markets come with their regulatory quirks. There’s also the prospect of aftermarket solutions, where older cars are retrofitted with autonomous features, thus expanding the technology’s reach. Unlike robotaxis, the scalability of autonomous passenger cars is influenced more by individual purchases than large-scale corporate fleet acquisitions. And it’s important to note that many AD benefits can be had even if only parts of the journey are automated.
Commercial vehicle automation might follow a corridor-based approach initially, focusing on specific high-traffic routes. As technology proves its mettle and regulatory frameworks adapt, broader national or even international routes might open up for automated commercial transit.
The autonomous driving landscape is diverse, with robotaxis, passenger cars, and heavy goods vehicles charting different paths toward a future of safer, more efficient, and convenient transportation. While their end goals align, the journey, influenced by varied motivations, challenges, costs, and scalability factors, is unique for each.
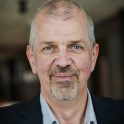
Jonas Ekmark
Head of Technology and Collaboration
Jonas Ekmark leads the team that provides knowledge and technology for Zenseact’s strategic needs through research, proof of concepts, and partnerships. He has prior experience with SAAB Automobile, Volvo Cars, and Zenuity. Since 1985, he has focused on developing technology to protect humans from losses in the road traffic system.